LSP Research Projects
Our research brings together diverse teams of physicians and scientists to solve the toughest interdisciplinary challenges in medicine. While our research projects cover a wide range of topics, they each apply innovative quantitative methods that enable a deeper understanding of biology. The primary goal of the lab is breakthrough research, but we also invest heavily in the development of software and fundamentally new approaches to pre-clinical and clinical pharmacology that enable novel insights.
Our projects are deeply collaborative, with several funded by multi-institution "Center" grants. Click on the "Learn more" links below to view any associated Research Centers and a list of selected publications.
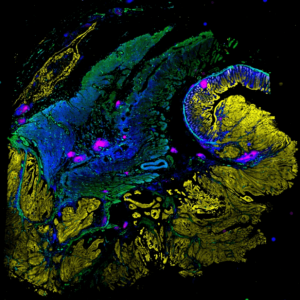
Developing next generation diagnostics and immunoprofiling methods using digital pathology and AI
The LSP has developed new highly multiplexed imaging methods and computational tools that enable deep molecular interrogation of tumor-immune interactions and the development of next-generation diagnostics for precision cancer care.
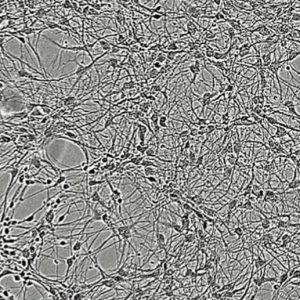
Repurposing drugs to treat Alzheimer’s Disease
LSP investigators working with the Massachusetts Alzheimer's Disease Research Center at the MGH are developing new approaches to treat Alzheimer's Disease based on the hypothesis that the disease has multiple distinct etiologies, some involving degeneration-associated chronic inflammation.
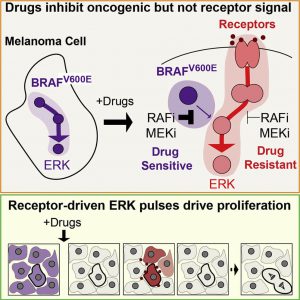
Identifying the determinants of sensitivity and resistance to small molecule kinase inhibitors
Kinase inhibitors are an intensively studied class of therapeutics with many remaining unknowns – LSP investigators use them to develop new approaches for identifying targets and to study the factors determining drug resistance and sensitivity.
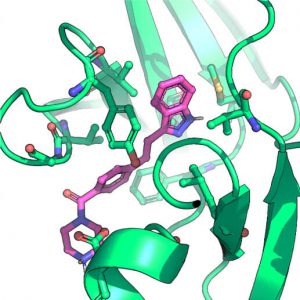
Using deep learning to model drug-target engagement
LSP investigators are contributing to and benefiting from the rapid advances in deep learning that are revolutionizing data analysis, protein structure prediction, and our ability to invent new drugs.
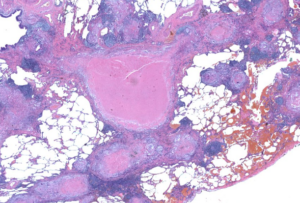
Developing Cures for Tuberculosis
Through a close collaboration with Tufts Medical School, LSP scientists are using computational approaches, animal models, and deep profiling of TB granulomas to understand how mechanisms of combination therapy and improve treatment approaches.
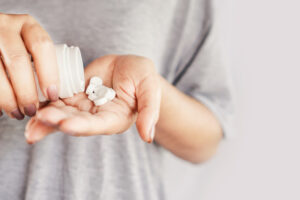
Identifying New Non-Opioid Therapeutics for Pain
LSP investigators are developing novel non-opioid treatments for pain with greater long-term efficacy and lower potential for abuse.
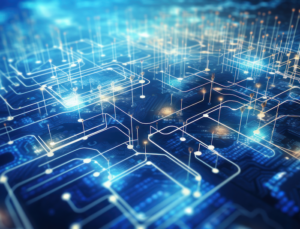
Using Knowledge Assembly to Enable Network Biology
The LSP is developing new knowledge assembly systems to dramatically improve how we extract causal and mechanistic information from published literature.
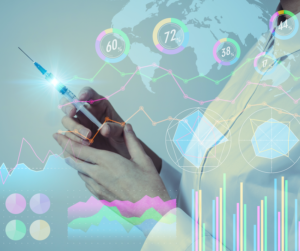
Improving the performance and interpretation of cancer trials
The LSP is engaged in a wide-ranging project to understand the causes of success and failure in cancer clinical trials and develop improved computational methods for early-phase trials that can help predict trial success.