Featured Member: Dan Freeman discusses how industry experience helped guide him to the LSP
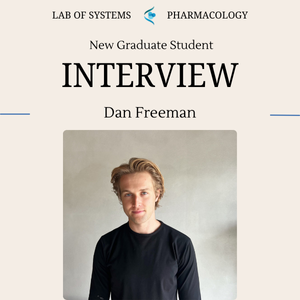
First year graduate student Dan Freeman’s journey to the LSP has been anything but linear, shaped by a series of unique experiences and motivations. A passion project in coding led him to explore novel technologies and entrepreneurship through his startup terraFlow, where he began the work that would ultimately guide him to Harvard and the Lab of Systems Pharmacology (LSP).
Dan’s focus on improving workflows through new technology and methodologies is shared by his advisor, LSP Director Peter Sorger, whose lab brings together researchers with diverse backgrounds and experiences. In his current project, Dan develops interpretable AI models for histopathology analysis. His work builds on OMERO, an LSP-developed platform that enables researchers to perform H&E and 20-plex immunofluorescent imaging on the same slide. Since H&E models often miss what’s happening on the molecular level, Dan leverages this additional data to guide models towards learning more reliable and meaningful features. He demonstrated this capability by teaching a model to detect mitotic cells known to drive progression and recurrence in melanoma.
As Dan continues working to advance medical science, the LSP provides an excellent ecosystem to test his ideas and collaborate with like-minded peers.
We sat down with Dan to hear about his work so far in the LSP, what brought him here, and where he envisions his work going. This interview has been condensed and edited for clarity.
What have you enjoyed most about your first few months at the LSP?
I feel very satisfied being a part of the team here. To me, science is about making the case for why your hypothesis, your diagnosis, or your course of treatment is correct; I’d say that is the unifying theme of my research and company. I want to understand the way pathologists think about cases and build on that in a meaningful way. AI is good at making accurate predictions, but I think the value of AI is turning AI outputs into something useful to a biologist or clinician. I see this notion reflected in the LSP, where people are set on developing new tools and methodologies to plug into existing technical workflows.
While I feel good about carving out a little piece of that to work for myself, I like knowing that I am still contributing to a larger goal. Much of the lab's work is geared towards an overarching vision of how different technologies will come together in the next 5 years, towards big ideas that can make a serious impact in medicine. That dynamic is why Sandro sits in on every lab meeting and gives comments from the perspective of a pathologist. There aren’t a lot of labs that work that way! I feel like the LSP is the perfect place for me to dig into this relationship between technology and medicine.
What path led you to the LSP?
Well, my original plan was to go to medical school. I’m the child of Russian and Israeli immigrants, so my options were either medical or law school, and my sister already took the latter. I was close to going – I had taken the MCAT and written the essays – but then I started teaching myself how to code. I ended up falling into the “AI/LLM world.” I knew I wanted to take this idea further, so I withdrew my medical school applications and joined the Precision Immunology incubator at NYU. There I began working on my start-up terraFlow and doing the work that led me to Harvard.
After the Incubator, I applied to graduate schools, was accepted to Harvard, and rotated through a couple of labs before coming to the LSP. I happened to be working in another lab that was collaborating with Peter and was motivated to join his lab. Anyone can talk about wanting to cure cancer or solve a disease, but the LSP seemed determined to move towards actionable intentions, with a game plan for their implementation. The desire to have a legitimate impact was something I prioritized for a long time when I started my PhD program.
What are you hoping to achieve in the future?
Prior to coming to Harvard, I worked alongside pharmaceutical companies to help run their clinical trials and discovered a huge bottleneck in how they analyze data. In an industry where robots process thousands of samples daily, analysis of clinical trial data is still often performed by individual scientists, who are at computers reading papers and testing hypotheses one at a time. I think the goal of AI should not be to replace these scientists but to provide them with better and faster access to information. Clinicians are using available information to layer on different types of evidence and arrive at a unique perspective; that is how they make their decisions. I want to create a tool that provides better information faster, leading to a more efficient process for scientists. This is really my ultimate goal – to understand what goes into the decision-making process and innovate from it.
Latest News
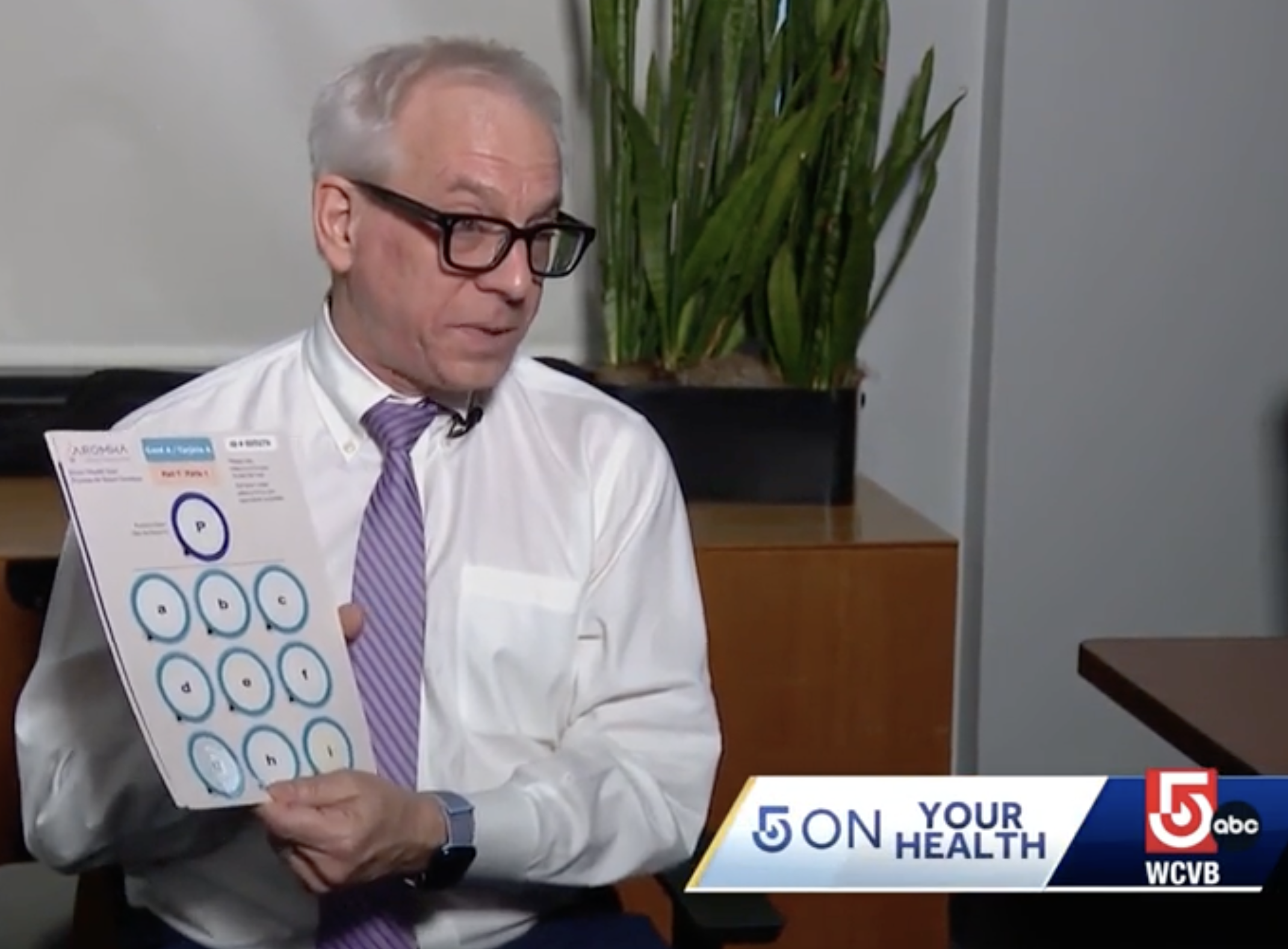
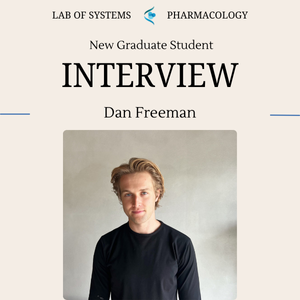
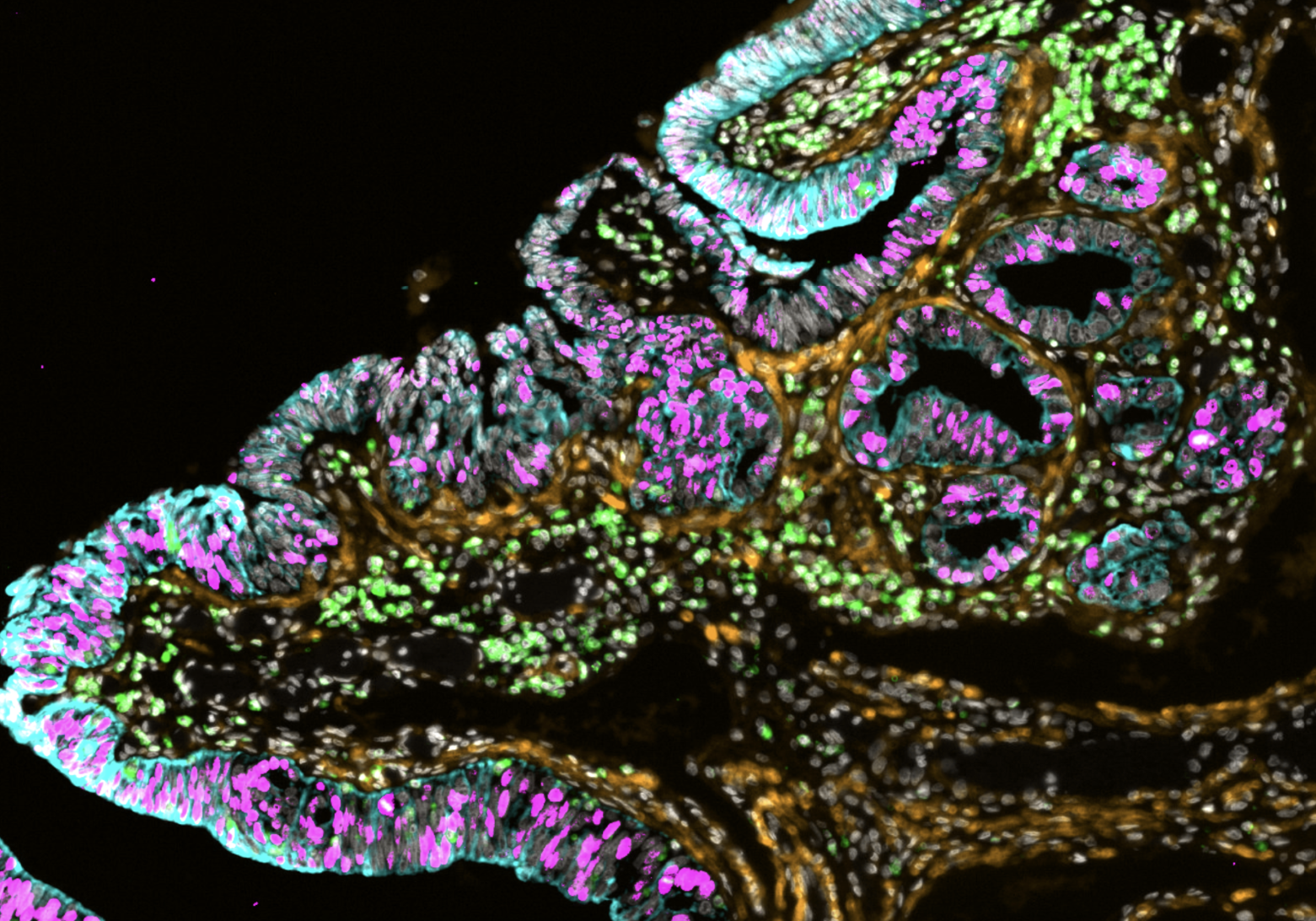
